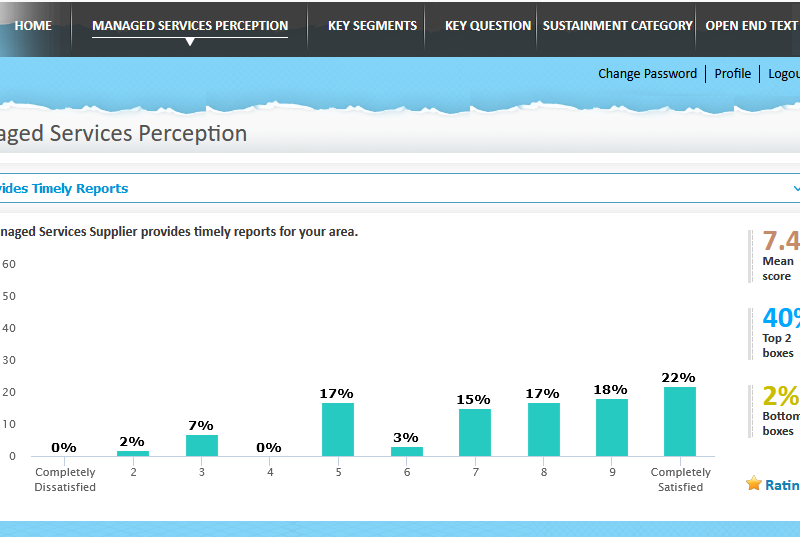
Marketers and researchers use the word ‘analytics’ to describe many different things that can be done with digital data. Without a common understanding, it can be easy to misinterpret what a client actually needs and end up assigning project tasks to the wrong people, costing jobs inaccurately, and not meeting client expectations.
In this post, we’ll take a deep dive into the different interpretations this word can have to ensure that both clients and suppliers are on the same page when it comes to extracting relevant insights from from myriad datasets about buyers, brands, and businesses.
Before we get into the details, you might appreciate this short introduction to data analytics from The Career Force on YouTube.
Types of Data
First of all, let’s look at some types of data that business leadership, marketers, brand managers, and researchers have access to in order to better understand consumer and market enigmas.
.
Primary research data
Primary research data is generally considered ‘small data. They’re easily stored in traditional spreadsheets like Excel and the files are small enough to be emailed without getting stuck in your outbox or flagged as spam. These data tend to represents people’s opinions and perceptions about various topics asked of them in a quantitative questionnaire, or a qualitative interview or focus group.
- Ad hoc survey or interview data: Often under 1000 records and under 100 variables. Normally focused on one brand or topic. Qualitative datasets converted to quantitative formats may have fewer records but much more, or much larger, variables.
- Tracker survey data: When gathered across multiple brands or countries, may be up to 50 000 records and a couple hundred variables. Normally focused on one product category though they may shift in focus from time to time.
.
Business data
Business data is often created in passing – as something happens in the company, a physical or digital record is created. Created and stored over years and in many disparate formats, these records are used to fulfill customer requests, manage employees, or keep track of product development. In many cases, these data are left lying around, ignored on servers, collecting virtual dust, and not leveraged for the insights that lie within.
- Employee data: Records of retention, satisfaction, reviews, salaries, promotions, complaints, departments and more can be transformed and standardized as variables for statistical analysis.
- Customer data: This is where we start to use the phrase “big data.” Transactional data reflecting purchases, SKUs, prices, times, dates, and more can come in datasets of millions or trillions of records with thousands of variables. Click-stream data gathered from websites can be exponentially more massive as every tiny movement and action made by a finger, pen, or mouse on digital screens is tracked. These data are already collected in standardized datasets and ready to be reformatted or transformed into specialized datasets for analysis.
- Business data: Executives are often most interested in these data – revenue, costs, finances, operations, inventory, supply chain, & logistical data. These data, also usually available in standardized datasets, are often summarized from individual level data but are even more valuable at the individual level.
.
Secondary research data
Secondary research data is all-encompassing. It can include any type of primary research or business data that were collected for some other purpose, whether by yourself, someone else at your company, or someone at a different company. As such, you might have access to small survey datasets, massive transactional datasets, or compiled and summarized datasets. In addition to the primary and research data already described, it could include:
- Third party data: A huge range of data types and sizes can be purchased from third parties that create, curate, and collate many sources of data, potentially terabytes of individual or summary level data.
- Social media data: Originally created to communicate a specific message to a specific person (or persons), social media data can be gathered and used for purposes other than originally intended. These data may include information about brands, people, and companies, date, time, geography, sentiment, and more. It may need to be transformed and standardized but a wealth of insights exist here as well.
Types of Analyses
There are three categories of analytics and skill-sets that might be required in the course of a research project.
.
Standard analytics
Most quantitative market researchers have a broad understanding of the theory and application of statistics. They know when and why to apply certain types of analyses to achieve specific research goals. Specifically, they have a lot of experience interpreting massive data tabulation files and running standard survey analyses to help us identify patterns and understand what happened and why.
They focus mostly on:
- Types of data: Primary data, usually quantitative survey data
- Types of analyses: Correlations, t-tests, chi-square, means, standard deviations, ANOVAs, descriptive and diagnostic statistics
- Analysis tools: Menu driven SPSS, Excel, data tabulations
- Outputs: PPT reports, static Excel reports
.
Questions to Find Out If This Is The Goal
- Will the analyses focus on details from the data tabulations?
- Do you need insights beyond what is covered in the data tabulations?
- Do you need anything beyond descriptive statistics like means, standard deviations, and box scores?
.
Possible Research Questions
- What parts of the shopper experience do my customers like the most?
- What problems does my product help consumers solve?
- What types or segments of shoppers prefer my brand and how can I tailor my products and marketing for them?
- How many people are aware of my brand and where does it sit in the competitive brand space? – A case study
.
Advanced analytics
Advanced analytics are usually conducted by people who have specialized training and expertise in statistics. They are experienced with non-standard and special cases of statistical tests that can’t be determined from data tabulations. Advanced analytics can help us understand what happened, why it happened, and predict what is likely to happen next.
They focus mostly on:
- Types of data: Primary research data, small business datasets, biometrics data
- Types of analyses: All of the standard analytics plus linear / logistic / multiple regression, conjoint, MaxDiff, TURF, factor analysis, cluster analysis, segmentation, discriminant analysis, perceptual mapping, special cases of standard analytics, predictive analytics, forecasting, and more
- Analysis tools: Menu or script driven SPSS, SAS, R, Python
- Outputs: PPT reports, static or dynamic Excel reports, user-guided dashboards, simulators
.
Questions to Find Out If This Is The Goal
- Do you need to segment people or products into groups?
- Do you need to predict purchases or forecast sales?
.
Possible Research Questions
- What is the highest price we can charge without making our customers feel ripped off while also earning as much profit as possible?
- What do my customers’ spending patterns look like?
- What is the best set of flavors for my products that won’t result in cannibalization? – A case study
- What is the potential size of my market given various product feature scenarios?
- Which employees are most likely to stay with our company for a long timer, thereby reducing costs of recruiting and training?
.
Business Analytics/Intelligence
Answering business intelligence questions to improve strategic decision making and create a competitive advantage normally requires advanced expertise in both statistics and data management. That skill set is often described as data science. Of course, for maximum effectiveness, you would also want this person to have extensive experience with marketing and consumer data.
These experts focus mostly on:
- Types of data: Big data, business data, transactional data, logistics, employee data, real-time or near-time data
- Types of analyses: All standard and advanced analytics, plus data transformation and manipulation, data fusion, data mining
- Analysis tools: Python, R, SAS, SQL, machine learning, AI
- Outputs: PPT reports, static or dynamic Excel reports, user-guided dashboards, simulators
.
Questions to Find Out If This Is The Goal
- Do you need to combine different types of data from multiple sources?
- Do you need to make sales or logistics predictions in real-time?
.
Possible Research Questions
- Why are we unable to keep warehouses stocked with the right products at the right time?
- Where are we dropping the ball with our processes and logistics, and how can we solve small problems before they become big problems?
- How can we increase our efficiency to improve our overall profitability?
- When a customer has selected a single product, what other products would they be most interested in?
- Can we drop a rarely purchased product without causing our highest value customers to switch retailers?
- How can we ensure optimal inventory for every SKU using existing business data? – A case study
.
What’s Next?
There’s a lot of overlap among various analytical techniques and objectives. One project may require only standard analytics whereas another may require all of them. However, once the research problem and the available datasets are clearly defined (not as easy as you’d think!), your analyst will know which techniques and software are best suited to uncover your answers.
If you’re ready to gather top quality insights about your buyers, brands, and business, please do email your project specifications to our research experts using Projects at E2Eresearch dot com. We’d love to help you turn your enigma into enlightenment!
Podcasts about Business Intelligence
- Analytics Power Hour with Michael Helbling, Tim Wilson, and Moe Kiss, Apple Podcasts
- Analytics on Fire with Mico Yuk, PlayerFM
- BIFocal – Clarifying Business Intelligence, Spotify
- Data Science at Home with Francesco Gadaleta, Spotify
- Data Stories: A podcast on data visualization with Enrico Bertini and Moritz Stefaner, Spotify
- Hub & Spoken: Data | Analytics | Chief Data Officer | CDO | Data Strategy, PlayerFM
- Making Data Simple by IBM, Apple Podcasts
- Raw Data By P3 Adaptive with Rob Collie, PlayerFM
- The PolicyViz Podcast with Jon Schwabish, Apple Podcasts
Business Intelligence and Data Analytics Conferences
- SAS Hackathon: Winter, 2022
- The Global CDAO: Driving a Global D&A Strategy: Beverly Hills, January 30 to February 1, 2022
- Gartner Data & Analytics Summit: Florida, March 14 to 17, 2022
- Big Data & Analytics Summit: Toronto, March 22 to 23, 2022
- Informs Business Analytics Conference: Texas, April 3 to 5, 2022
- CIO’s Data & Analytics Summit: April 13 to 15, 2021
- SIAM International Conference on Data Mining (SDM22): Virginia, April 28 to 30, 2022
- Data Innovation Summit 2021: Stockholm, May 5 to 6, 2022
- TDWI Data Conference: Chicago, May 8 to 13, 2022
- Data Summit Connect: Boston, May 17 to 18, 2022
- SAS Innovate: San Diego, April 11 to 13
- TIBCO Analytics Forum: June 13 to 15, 2022
- DATA 2022: Lisbon, July 11 to 13, 2022
- SIAM Annual Meeting (AN22): Pennsylvania, July 11 to 15, 2022
- Industrial Conference on Data Mining (icdm): New York, July 13 to 17, 2022
- Real Business Intelligence: September
- SAS Explore: Fall, virtual
- Chief Data & Analytics Officers Exchange: September
- The 8th IEEE International Conference on Data Science and Advanced Analytics: Portugal, October 6 to 9, 2021
- Chief Data & Analytics Officers (CDAO), October 12 to 14, 2021
- BigData & AI: Toronto, October 13 to 14, 2021
- The Data Science Conference: Boston, October 14 to 15, 2021
- DataScienceGO: California, October 15 to 17, 2021
- Open Source Analytics (OSA CON): November 2, 2021
- Marketing Analytics and Data Science: Tennessee, November 15 to 17 2021
- Business Intelligence & Analytics Conference, London, November 14 to 17, 2022
- Disney Data & Analytics Conference: Florida, November 30 to December 1, 2021